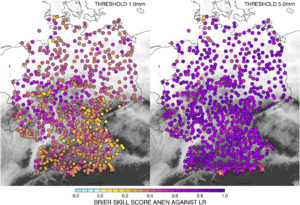
Post-processing in weather and climate is aimed at enhancing the output of meterological models by applying a statistical modeling framework using the simulated estimates together with observations in a training data set. These methods are often used to enhance weather forecasts by accounting for the systematic errors in the model output. Post-processing approaches can also be applied to climatological model data, i.e., reanalyses, to bring the estimates closer to the truth or to increase the targeted resolution such as in statistical downscaling approaches.
One aspect we focus on is the development of probabilistic post-processing of reanalysis data. Such a method cannot only provide improved estimates of relevant meteorological parameters like wind gusts or precipitation, but also enable the assessment of the uncertainty which is of great importance for risk assessment, e.g., in the field of renewable energy.
Examples for current developments are
- Downscaling post-processing scheme of regional reanalyses using the analog ensemble approach
- Multi-method post-processing of weather forecasts aimed at radar data with classical compared to neural network (AI) approaches
- Probabilistic post-processing for the spatial distribution of wind gusts (e.g. gusts at different vertical heights or over extended spatial areas).